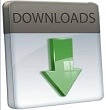
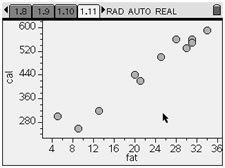
In this graph, you can see that the distribution of the variable on the X axis (horizontal) is right skewed while the distribution for the variable on the Y axis (vertical) is fairly symmetrical. This type of plot simply graphs the distribution of each of the variables in a scatterplot separately in the margins, as shown in the example below. However, you can assess the distribution of values for individual variables in the context of a scatterplot by using a marginal plot. The skew of a distribution relates to the distribution of a single variable, and you should use a histogram for that. Scatterplots highlight relationships between pairs of variables. When you’re looking at pairs of values as you’re doing in a scatterplot, terms like skew of distribution don’t make sense. They also account for the possibility of random error explaining the observed patterns and differences.Ĭorrelation and regression analysis are the primary methods for statistically assessing relationships between continuous data. On the other hand, hypothesis tests present an objective evaluation of statistical significance. Altering these settings can change the appearance of scatterplots and the conclusions you draw from them.
#Scatter plot examples real life software
Graphs can be subjective because your software lets you edit their properties, such as the graph’s scaling. Related post: Descriptive versus Inferential Statistics However, if you plan to use your sample to infer the characteristics of an entire population, be sure to perform the necessary hypothesis tests and assess statistical significance. You can use scatterplots to display the relationships between continuous variables. When creating a scatterplot for time data, be sure to add a connect line between the data points! Use Scatterplots with the Appropriate Hypothesis Tests Scatterplots are a perfect choice for time-related data when your observations occur at irregular intervals. However, you can also use scatterplots for this purpose.
#Scatter plot examples real life series
Typically, analysts use time series plots to display data over time. Related post: Five Ways to Find Outliers in Your Data Trends Over Time However, the combination of the two values clearly does not fit the overall relationship. In the scatterplot below, the circled point has X and Y values that are not unusual. Unusual observations have values that are not necessarily extreme, but they do not fit the observed relationship. These outliers are distanced from other data points, as shown below. Scatterplots can help you find multiple types of outliers. Related post: Comparing Regression Lines with Hypothesis Tests Find Outliers and Unusual Observations with Scatterplots Use indicator variables and interaction terms in a regression model to test the statistical significance of these differences. As the input value increases, the output for group B increase more quickly than group A. In this scatterplot, the slope for group B is steeper than for group A. In this scatterplot, the slope of the relationship is the same for the two groups, but the output values of group B are consistently higher for any given input value. All groups must use the same X and Y measurements. To make these comparisons, you’ll need a categorical variable that defines the groups. When your data have groups, you can determine whether the relationship between two variables differs between the groups. Related post: Modeling Curvature Using Regression Determine Whether the Relationship Changes between Groups When a relationship exists, you might want to model it using regression analysis. When a relationship between two variables is curved, it affects the type of correlation you can use to assess its strength and how you can model it using regression analysis.Īdding a fit line highlights how well the model fits your data. Related post: Interpreting Correlation Coefficients Linear and Curved Relationshipsĭetermine whether your data have a linear or curved relationship. Stronger relationships produce correlation coefficients closer to -1 and +1 and regression models that have higher R-squared values.
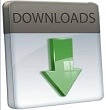